Reasoning Models, A New Era of Explainable AI
Explainable AI (XAI) has long been a critical area of research, striving to shed light on the decision-making processes of increasingly complex AI systems. Understanding how an AI arrives at a particular conclusion is crucial for trust, accountability, and identifying potential biases. Traditionally, XAI has relied on techniques that either utilize inherently transparent models or treat the AI as a "black box," offering limited insight into its internal workings.
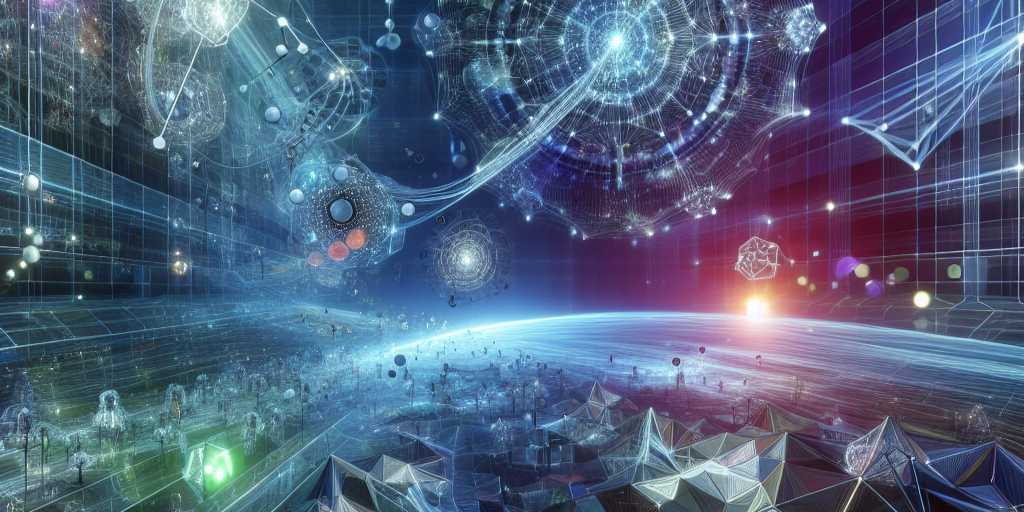
However, a new class of models, known as reasoning models, is emerging, promising a potential revolution in XAI by offering inherent transparency into their thinking
process. This post explores how reasoning models, such as Deepseek R1, OpenAI o3, and Google Gemini-2.0, are changing the landscape of XAI by exposing their reasoning steps and offering a more direct path to understanding AI-driven decisions.
Reasoning Models: Unlocking the Black Box of AI
Reasoning models represent a new approach to explainable AI (XAI). Unlike traditional XAI methods that rely on external techniques or model-specific architectures like decision trees, reasoning models, such as Deepseek R1, OpenAI o3, and Google Gemini-2.0, inherently expose their thinking
process. These large language models (LLMs) decompose complex questions into a "chain of thought," revealing the steps taken to arrive at a solution.
This transparency provides insight into the model's decision-making, aligning with the core goal of XAI: understanding how AI systems produce results. This shift towards inherent explainability within the model itself marks a significant departure from previous "black box" approaches and offers a potentially more generalizable solution for XAI.
From Black Boxes to Transparency: The Evolution of XAI
Explainable AI (XAI) aims to make AI decision-making processes understandable to humans. Traditionally, achieving explainability involved using inherently transparent models like decision trees. When more complex models were necessary, techniques like SHAP, which treat the model as a "black box," were employed. These methods provided insights into feature importance but didn't reveal the internal workings of the model itself.
Reasoning models, such as DeepSeek R-1, OpenAI's o1, and Google Gemini 2.0, represent a shift towards inherent transparency. These models expose their thinking
process, revealing the steps taken to arrive at a given result, thus providing a more direct form of XAI. This "chain of thought" approach allows users to understand not just what decision was made, but how the model arrived at that decision.
Conclusion
Reasoning models signify a potential paradigm shift in explainable AI. By exposing their internal thinking
process, these models offer a level of transparency previously unavailable with traditional black-box AI systems or methods relying on external explanation techniques. As discussed, the ability to observe the step-by-step reasoning chain provides valuable insights into how these models arrive at their conclusions.
This inherent explainability, exemplified by models like Deepseek R1, OpenAI o3, and Google Gemini-2.0, promises to foster greater trust and understanding in AI systems, paving the way for wider adoption and more responsible application across various domains. The shift towards inherent transparency in reasoning models represents a significant advancement in the ongoing evolution of XAI, moving away from post-hoc explanations and towards a future where understanding how an AI reaches a conclusion is as readily available as the conclusion itself.